Avoid Boiling the Ocean: Taking Baby Steps Towards a Data Quality Strategy
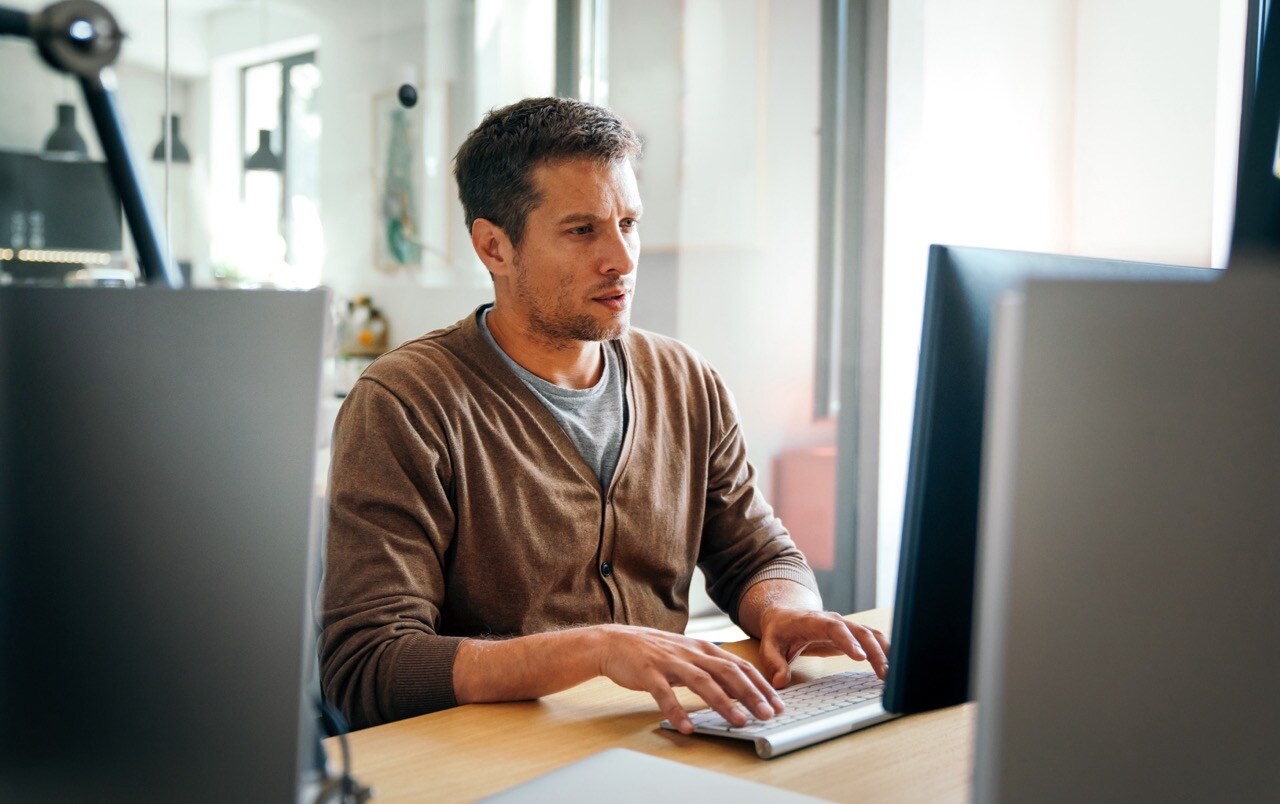
The poor confidence in analytical reports and data is so widespread that there is no point in pretending otherwise.
It’s so pervasive that over time we’re tempted to simply accept the status quo and avoid tackling the problem, fearful of an array of challenges such as:
- Fear of high cost: Because Data Quality projects can be ambitious, the fear of unpredictable implementation costs can be daunting.
- Fear of excessive Data Engineering staff utilization:
Data engineers are constantly busy implementing new requirements that arrive at the speed of light (along with challenging delivery timelines!) In this environment, it’s extremely difficult to take on a new initiative. - Fear of criticism and conflict: Data Quality issues revealed during discovery and profiling can lead to defensiveness within various departments and complicate relationships with external data providers. If the analysis reveals sufficiently complex processing problems, it may require a politically embarrassing code overhaul.
- Fear of tooling cost and complexity: Data quality infrastructure and data quality tool licensing can be costly. In addition, there is a fear of the learning curve eating into the bandwidth of Data Engineers.
However, the risks of poor Data Quality make it well worth addressing these fears. Attrition of customers due to lost confidence in data, growing frustration and dissatisfaction of burned out data engineers, lack of confidence in Decision Support Systems (DSS), integration complexities of downstream systems are just a few of the inevitable outcomes directly or indirectly impacting the overall reputation of an organization.
Take baby steps
The best way to initiate a Data Quality strategy is to start small. Perform a pilot of limited scope, measure the effectiveness, take actions and gradually mature. A typical maturity model can be:
Before kicking off the initiative, be sure to prepare yourself for the potential issues. Perhaps the biggest of these is ensuring consensus on the Goal of this initiative. Examples might be to bring down the cost of solving Data Quality tickets, or perhaps increase the effectiveness of Machine Learning models. By doing so, it will become clear which areas or data elements must take the highest priority. This is the stage of awareness, acceptance, and commitment to solve the problem. Define a clear roadmap to success and finalize the data quality software to be used.
Level-1
This is when the action starts. Key is to have very limited scope and focus on the low complexity and high value addition entities (which helps address the fear of excess bandwidth utilization). If cost is a major concern, then open source tools are the best bet (overcome fear of license cost). The majority of the data quality tools are bundled with a Monitoring Dashboard, leverage it. Perform a thorough Data profiling to extract the Data quality rules for monitoring.
It is important to identify the location within the pipeline to monitor the Data Quality metrics.
E.g., if the data is procured from a third party, then monitor the quality in the landing zone when the data arrives in your premises (e.g. folder, GCS bucket, s3 bucket, etc.).
If there is a major transformation stage, then perform the data quality checks against baseline, etc.
Identify Data Stewards accountable for the Data and leverage the Data Quality Dashboard Observability during weekly Data Quality review meetings. One positive side-effect outcome of this review board is a focus on cleansing the data, initiating a course of action which leads us to the next level of maturity- Level-2 Cleanse and Mature.
Level-2
Here is a sneak peek into the Executive Summary dashboard:
An operational dashboard can provide a granular view of Data Quality issues. Below is a sample report generated from an open source tool - Great Expectations.
The Level-2 Cleanse and Mature is fairly self explanatory and, by this stage, the value generated by the Data Quality project is obvious to all. It is important to identify an effective location within the pipeline to perform cleansing operations. Note that there are different recipes to perform cleansing which depends on data flow or the workflow.
Be aware that Data Quality fixes or cleansing costs increase even more than data moves to the downstream stages.
Once the fears are addressed and a comfort zone is established, follow the Shewart cycle pattern of Plan-Do-Check-Act throughout the life of the Data Quality Strategy.
A further step in the logical progression towards maturity is the integration of incident management, such as warning/error alerts and automation of alternate paths in the data flow based on Data Quality results in real time.
So let’s revisit the fears we talked about at the beginning of this post. By implementing the approaches outlined above, and following a few tips listed here, there’s no need to let fears prevent you from reaping the benefits of a solid Data Quality program.
Mitigating the Fear of high cost:
The metrics collected enable you to perform a simple cost analysis of fixing Data Quality tickets raised by your data consumers. In addition, a quantification of a missed CSAT score can add fuel to fire. Outcome of such cost analyses provides clarity and motivating factors for a Data Quality Strategy.
Mitigating the fear of excessive Data Engineering staff utilization:
Perform a comparative study by measuring the bandwidth spent by Data Engineers in resolving Data Quality issues reported by the customers pre and post Data Quality project implementation. A Data Quality initiative recoups that wasted bandwidth, encourages better coding discipline, and increases the confidence of Data Engineers.
Mitigating the fear of criticism and conflict:
Be sure to emphasize that the goal of analysis is mutual improvement and benefit, not blame. Setting metrics-driven Data Quality project goals will help gain the confidence of relevant teams.
Mitigating the fear of tooling cost and complexity:
We need to be cognizant of the fact that Data Quality is an essential constituent of any Data Engineering profile, and these skills bring long-term benefits from the investment. Further, Data Quality efforts bring measurable overall cost benefits when considering the savings from reduced manual correction, errors, and confusion.
Conclusion
Following a pilot framework eases the anxiety of initiating a Data Quality project. The importance is even higher for a Data driven organization and having Data Quality as an afterthought may have negative business implications.
At Pythian, we can ease the adoption of Data Quality initiatives through our highly flexible, cloud-agnostic Data Quality Accelerator enabled using Open Source Technologies. Our Data Quality Maturity Model provides a flexible roadmap that enables you to scope your Data elements and begin witnessing the results in less time than you may have imagined...
We are here and happy to help.
Share this
You May Also Like
These Related Stories
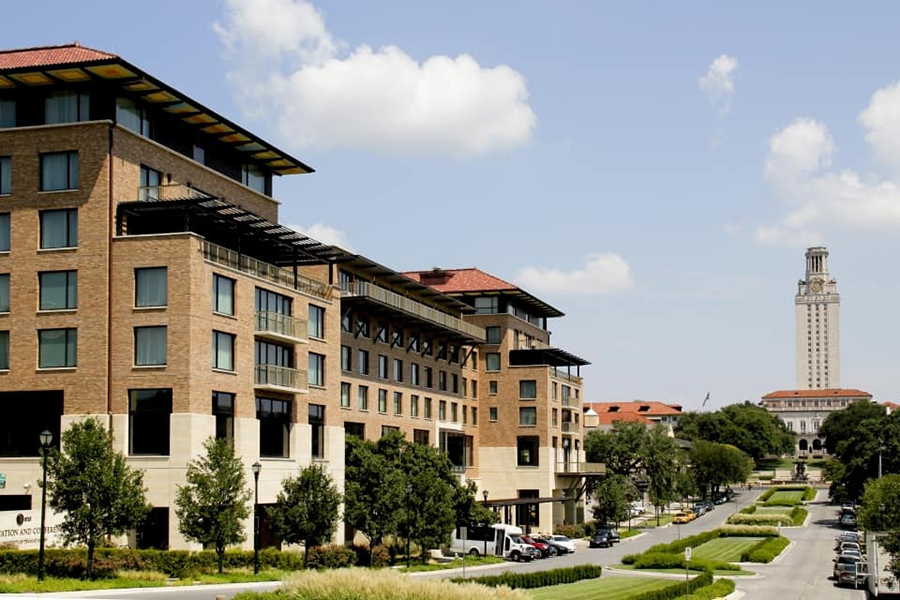
Data Day Texas 2024 Recap
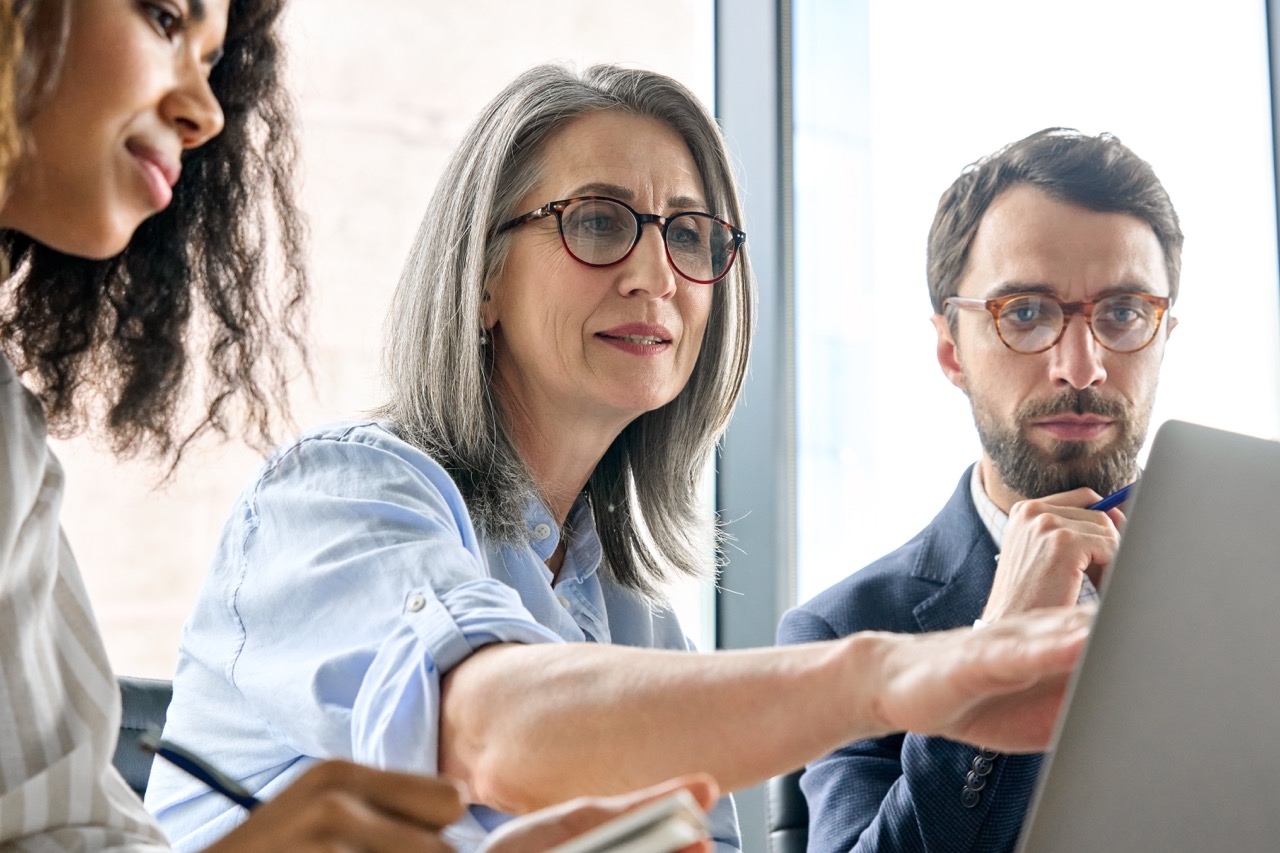
Data Strategy & Digital Transformation

No Comments Yet
Let us know what you think