How Marketers Can Leverage Google Cloud ML to Model Total Customer Lifetime Value
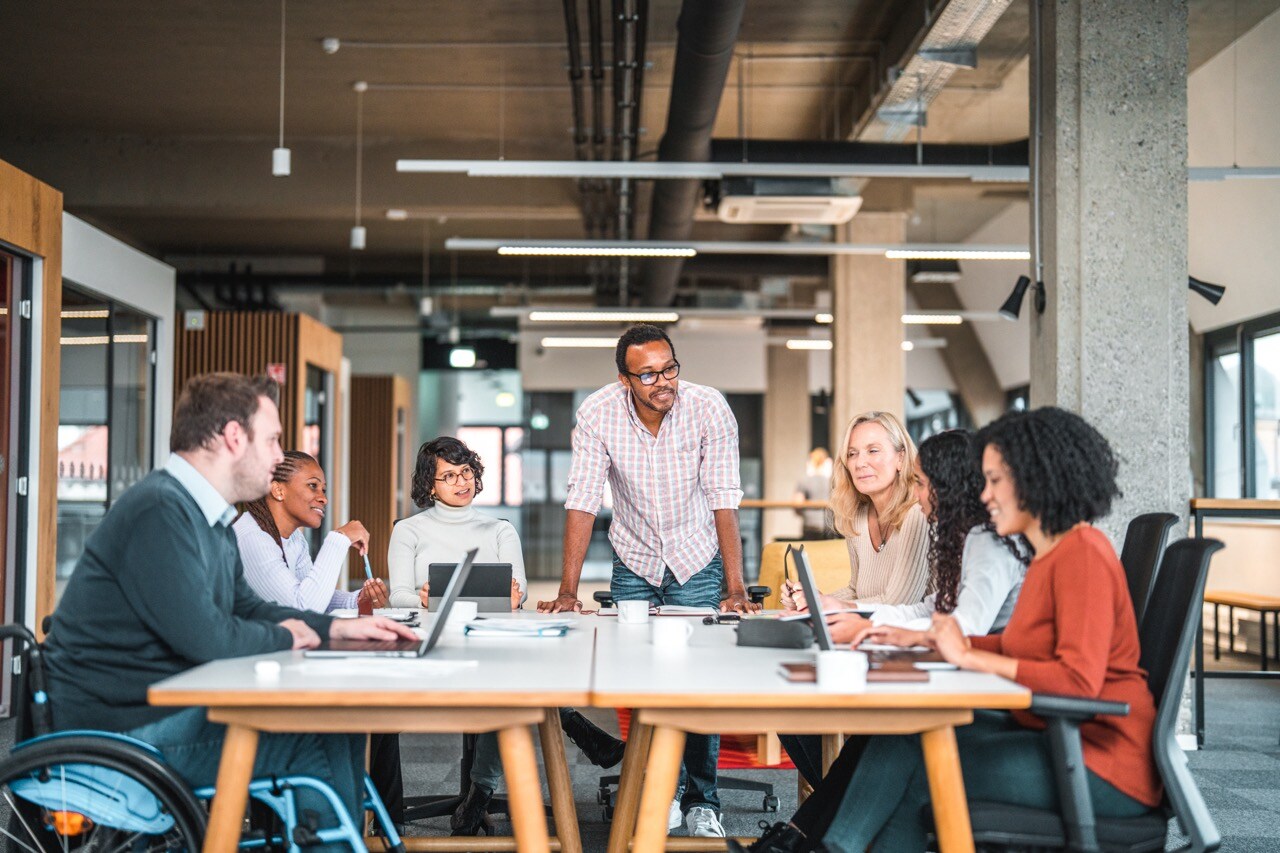
Marketers know that the key to success is to focus on customer experience. But to truly be customer-centric at scale, marketers also need to be data-driven.
Understanding Customer Lifetime Value (CLV) can help to create a ‘roadmap’ of how much a customer is likely to spend over their expected lifetime, which can inform marketing investments based on current and predicted performance and inform the overall marketing strategy.
CLV can also be a good metric to assess the health of your business—for example, by seeing if once-loyal customers are spending less money with your business over time. This data can be used to better predict your churn rate so you can adjust course if necessary. It can also help you understand which customers are most valuable and manage resources accordingly.
Marketers can also use CLV to strategize on marketing for different customer-value segments. This type of modeling can answer a variety of questions:
- How many purchases will a customer make in a given period in the future?
- How long will a given customer remain active?
- How much value will a given customer generate in a given period in the future?
This feeds a company’s ability to make informed decisions, such as:
- How much should we spend on advertising, and in which areas?
- Which specific customers should we target with different types of marketing?
- With customers segmented according to spending habits, how can we move customers from one segment to another?
How to leverage your data
There are several methods for calculating CLV, depending on your business. While purely statistical approaches can work, often machine learning (ML) approaches give the best results. A canonical approach uses historical information on customer behavior (such as purchase information, recency, and frequency) and demographic information as model inputs with either number of purchases or dollar value generated over a fixed timeframe as a regression target, or binned number of purchases or value generated as a categorical target.
Regardless of the method you choose, you need high-quality data for model training—to predict the future value of existing customers as well as new customers you’d like to nurture long-term.
But building, testing, and deploying analytical models is a lot of work—especially since these models need to be continually tweaked and adjusted. Google Cloud can do the heavy lifting for you thanks to built-in capabilities that leverage your existing data. For example, with Google’s machine learning tools, you can model CLV using data and a robust predictive analytics toolset to estimate the total value a customer is expected to generate.
Google’s Marketing Platform includes Google Analytics 4, its next-generation of analytics, which replaces Universal Analytics 360. Google Analytics 4 collects event-based data (rather than session-based data) from websites and apps to help you better understand the customer journey. It also includes predictive capabilities and direct integrations to media platforms. BigQuery ML allows you to create and run ML models and perform AI tasks. These tools can be key differentiators in achieving your marketing analytics goals.
Here’s a high-level step-by-step guide on how to leverage ML to model CLV:
Consolidate customer data: Gather data from various sources such as transaction history, demographic information, and customer interactions. Then, integrate this data using Google Cloud tools like Cloud Storage, BigQuery, and Dataflow.
Clean and pre-process data: Use tools like Dataprep or Dataflow to clean and transform data into a format suitable for machine learning. Handle missing values and outliers to standardize data across features.
Create relevant features: Extract meaningful features from the data that can influence CLV, such as purchase frequency, average transaction value, and recency of interactions.
Define the target variable: Label your dataset with the target variable—that is, the value you want your ML model to predict. In this case, we’re looking at CLV, but ML models can be used to investigate countless other values and data points. For CLV, the target variable is usually the purchase amount or value in a given time period.
Choose a machine learning algorithm: Depending on the nature of your data, consider using either regression models or binning techniques along with classification models. Strong choices for ML models include linear regression, decision trees, neural networks, and ensemble methods. Google Cloud has a suite of tools like BigQuery ML, Vertex AI Platform, and AutoML Tables for model development.
Train the model: Divide your dataset into training and testing sets; then, use the training set to train your ML model. Google Cloud’s Vertex AI Platform can be used for distributed training on large datasets.
Optimize model performance: Use tools like Vertex AI Platform Hyperparameter Tuning to automatically search for the best hyperparameters, enhancing the performance of your CLV model.
Evaluate model performance: Assess your model’s performance on the testing set using metrics like Root Mean Squared Error, or other relevant evaluation metrics for classification models.
Deploy the model: Once you’re satisfied with the model’s performance, deploy it using Vertex AI Platform Prediction for real-time predictions or batch predictions on new data.
Monitor model performance: Implement monitoring solutions to track the model’s performance over time. Regularly retrain the model with updated data to ensure its relevance.
Integrate CLV predictions into business processes: Use the CLV predictions to inform marketing strategies, customer segmentation, and personalized campaigns.
Establish a feedback loop: Continuously refine and improve the model based on feedback and new data. This ensures that the CLV predictions remain accurate and reflective of changing customer behaviors.
By following these steps and leveraging Google Cloud’s machine learning capabilities, it’s possible to develop accurate, flexible Customer Lifetime Value models to inform strategic decision-making and enhance customer relationship management.
Need help turning your disparate data into a comprehensive marketing strategy? Pythian’s Google Cloud experts can help your team implement and build machine learning models to take your marketing analysis to the next level. Get in touch today!
Share this
You May Also Like
These Related Stories
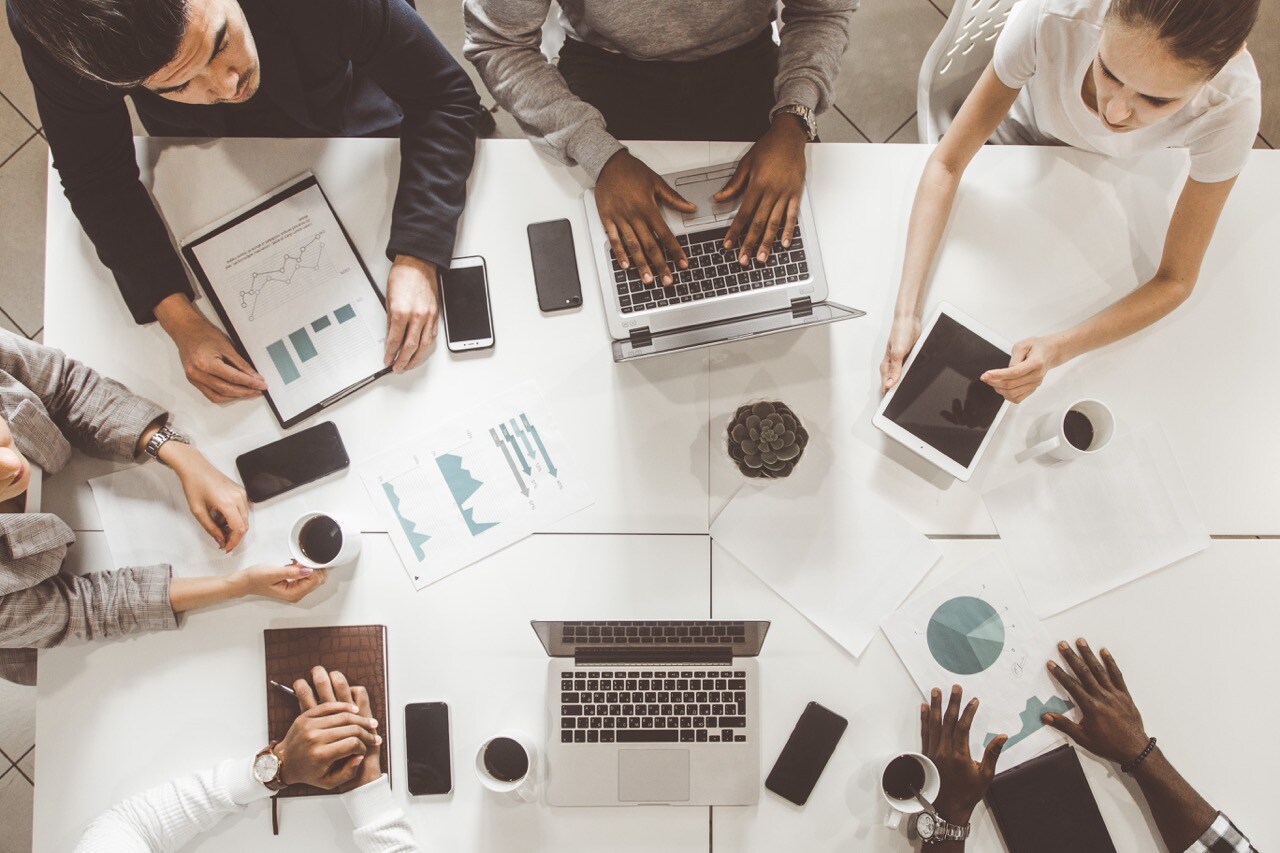
Accelerate Marketing Insights on Google Cloud with Pythian
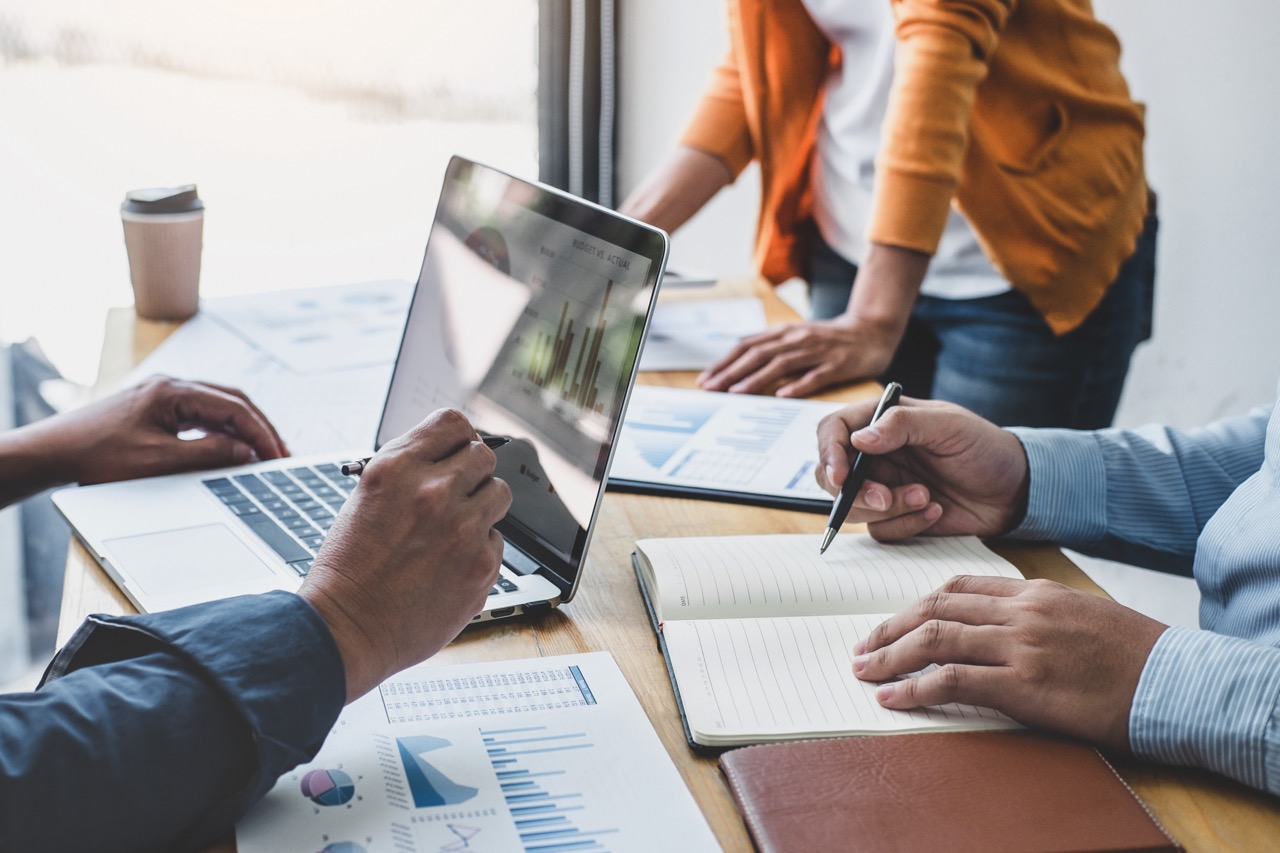
How to Improve Customer Segmentation Through Improved Data Quality
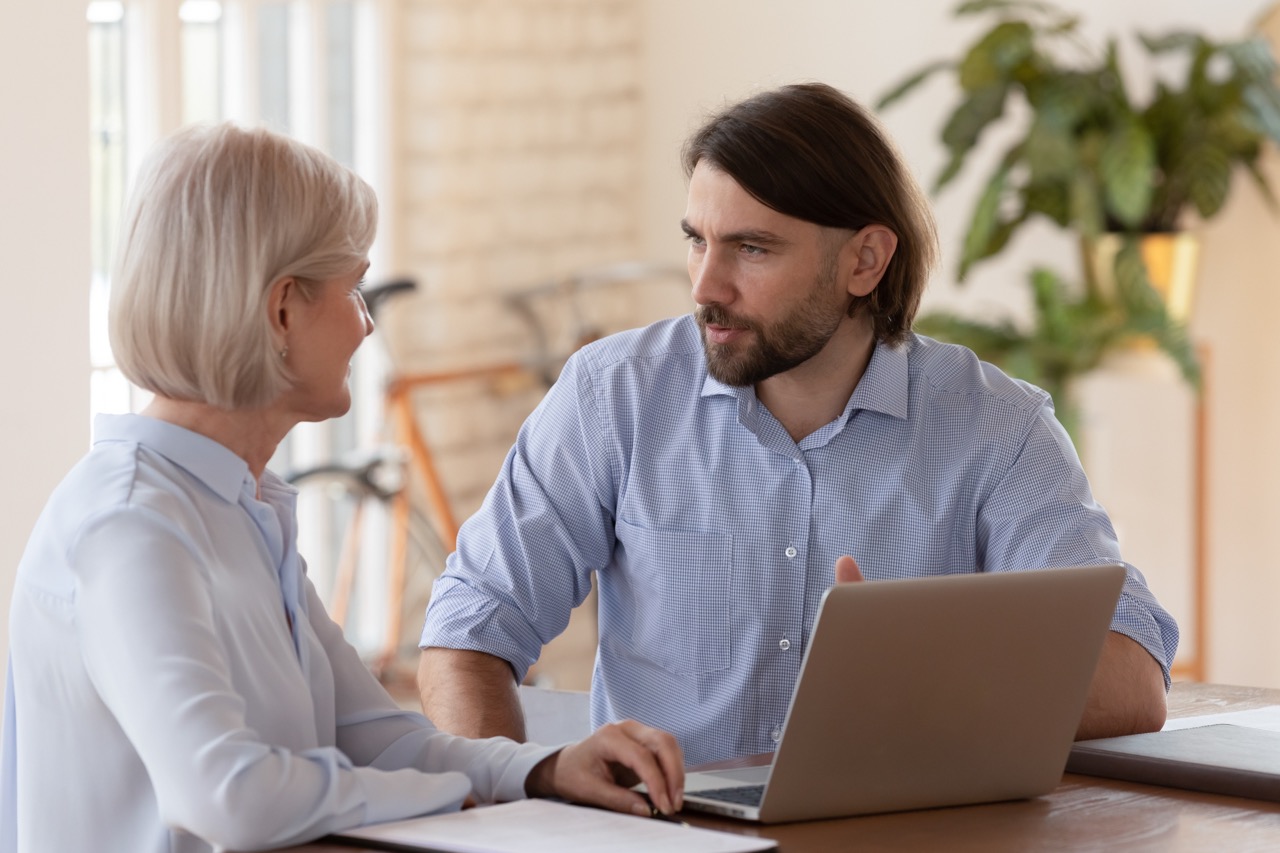
No Comments Yet
Let us know what you think