5 tips for businesses to build a strong relationship with data
A heart flutter. An adrenaline rush. The constant wondering of what will happen and where things will go. Wanting to spend more time, ask more questions and uncover new information to learn all you can.
These are the telltale signs of that heart-pounding, nervous excitement of working with data and analytics.
Treating your business’ data as if you’re starting a new relationship is what makes the difference between strategic transformation and directionless wandering.
And we don’t mean a glossy, stereotypical rom-com kind of a relationship (though, those can be fun too). We’re talking about the relationship that builds and deepens over time, that’s rooted in a drive to continuously understand and learn more. Because when it comes down to working with stakeholders across the company to align on a vision, cleaning and prepping data, and all the effort that makes data-backed transformations possible, a relationship with data takes commitment.
And in times like those, it can be easy to get distracted by the nitty-gritty, everyday challenges.
Wherever you and your company might be in your data and analytics journey, we’ve compiled five tips with insights from fellow data lovers to help keep the fire alive.
Tip 1: How to know if you’re ready to start or deepen your relationship with data
Many times, what gets discussed is what the data will help accomplish. Aligning on a vision and setting the strategy are all pivotally important, but they’re only half the equation. We also have to talk about how to make it possible.
Finding the one – or in this case, a team of them – is no small feat.
“Structuring a data science team depends on a number of factors, from the size of the organization and how centralized its analytics initiatives are, to its overall data strategy, objectives and budget,” writes Ronald van Loon, CEO, Principal Analyst Intelligent World, in “Tips for building a successful data science team: All you need to know.”
The types of results Ronald mentions can be big, small and everywhere in between – effectively scaling algorithms in production, identifying and solving the right business problems, and empowering stakeholders across the company through communication and more.
“Data science skills are frequently structured around a specific project or business question, and when there’s an understanding of how certain roles and responsibilities align with business objectives, it can help team members deliver a more successful result,” Ronald goes on.
Getting the right team or finding an outside consultant to support will create a strong foundation capable of blossoming into a transformational program.
Tip 2: First impressions aren’t everything
Raw sets of data are rarely perfect. Errors in collection, outliers, lack of variability and more can skew what we’re initially thinking the data is telling us.
It’s when we go a little deeper that we can see what it really means.
“3 steps for harnessing the power of data,” an article published by KD Nuggets, reminds us that first impressions aren’t everything.
Outliers are often just bad data, the article says, but simply omitting them could be too optimistic of an approach. Sometimes what they’re actually telling us is that there’s a malfunction in the system. And if it’s significant enough, it could completely throw off the predictive model’s power.
Missing data is also common, and often the easiest way to deal with it is by, again, omitting it. But then we lose out on all that important data. Instead, try different interpolation techniques. Replacing with mean, medium or mode (ahh classic statistics) could be the solution.
While the article goes on to cover other imperfections, we can start to see how even though numbers never lie, they sometimes are coy in what they’re actually communicating. Curiosity and a little bit of patience can help solve a lot.
Tip 3: Spend time with and put effort into your data
As you’re being a numbers sleuth (do you have that on your resume?), determining what coy numbers are actually trying to say, you’re ultimately spending a lot of quality time with your data.
In relationships with people and data, putting in the time and effort is worth it, especially when it comes to cleaning and preparation.
“It is not unusual for 90-95% of the time to be spent in data cleaning and prep,” writes Kirk Borne, Chief Science Officer at Data Prime, for Medium in “Data profiling – Having that first date with your data.”
But it’s not wasted time.
“On the contrary, this ‘date with your data’ is time ‘well invested,’” Kirk goes on. “You will develop greater confidence in and advocacy for your analytics project results by working with the cleanest possible data.”
By giving data our time, effort and thought, we’ll be rewarded later with knowledge and accuracy. We’ll be better equipped to share why the data says what it says – even if and especially when it’s not what was hoped for – because we’ll have done our due diligence.
Tip 4: Ask the right questions to create a deeper understanding
Questions are incredibly important. How they’re phrased, where the recipient is coming from (e.g., experiences, biases, etc.) and what you’re hoping to get out of them shape the outcome dramatically. And often, technical and business folks are credited with not always speaking the same language, so getting on the same page on what a specific model needs to do can be trying.
Dean Abbott, Chief Data Scientist at SmarterHQ, talks on an episode of the podcast Hidden in Plain Sight about how knowing what questions to ask, or asking enough questions to find the right ones, has helped him along significantly in his career. He says that by doing so you will uncover hidden assumptions and help procure the insights that a business leader is asking for from data.
He gives an example. A business leader wants to generate more ROI from an email campaign. That seems straightforward enough, but it’s also vague. At what cost do they want to generate ROI?
You might then ask that if the model generates a million dollars of revenue but also causes a 10% unsubscribe rate, would that still fit their vision?
If the response is something to the effect of it has to generate a lot of revenue and keep customers for the long haul, you learn that what’s truly needed is a model that performs in the near term while preserving long-term engagement. Your next question might then clarify how long term they’re envisioning.
Keep asking and you’ll eventually reach what becomes a shared vision. Then do the same thing once you have a model in its early stages of development. You’ll have something in practice and more tangible to discuss, which can uncover even more details and assumptions that weren’t apparent before. At the same time you’re doing all of this, you’re building mutual trust and a sense of collaboration that’ll bode well for future programs.
Tip 5: Introduce the friends and family
When any relationship reaches the stage of meeting loved ones, it can get nerve-wracking. It’s that time when loved ones want to see for themselves what you have to offer.
In the data world, other stakeholders across the company are the loved ones. And they want to see what the data has to offer.
For data programs to have successful longevity, employees with vested interest across the company need to be able to speak the language.
In an episode of The Data Chief podcast, hosted by Cindi Howson, Cindi speaks with Managing Executive Officer & CDO at Rakuten Takuya Kitagawa about Rakuten’s approach to cross-functional data fluency.
Regular meetings dedicated to the company’s various data and analytics programs bring together executives from across the company. Before COVID-19, they were quarterly, full-day, in-person bootcamps, but the company has since transitioned to doing them virtually every two weeks for 30 minutes. Required training courses and continuing education opportunities are other options to consider.
This ongoing dedication to and education on real-world work helps employees understand what’s possible, what things mean and generally be proficient in how to use data to move the business forward. So when the time comes for data and analytics conversations, everyone is coming in with the same baseline with the opportunity to accelerate from there.
Stoking the fire of data passion
Building your relationship with data doesn’t need to be a shout-it-from-the-rooftops kind of love (if that’s where you’re at, though, you might see us up there too). What will go a long way are healthy doses of curiosity and persistence, and you will be on your way to nurturing a strong relationship. Not sure where you’re at in your data love story? Take this quick analytics quiz to find out.
Share this
You May Also Like
These Related Stories
Data is the heart of business
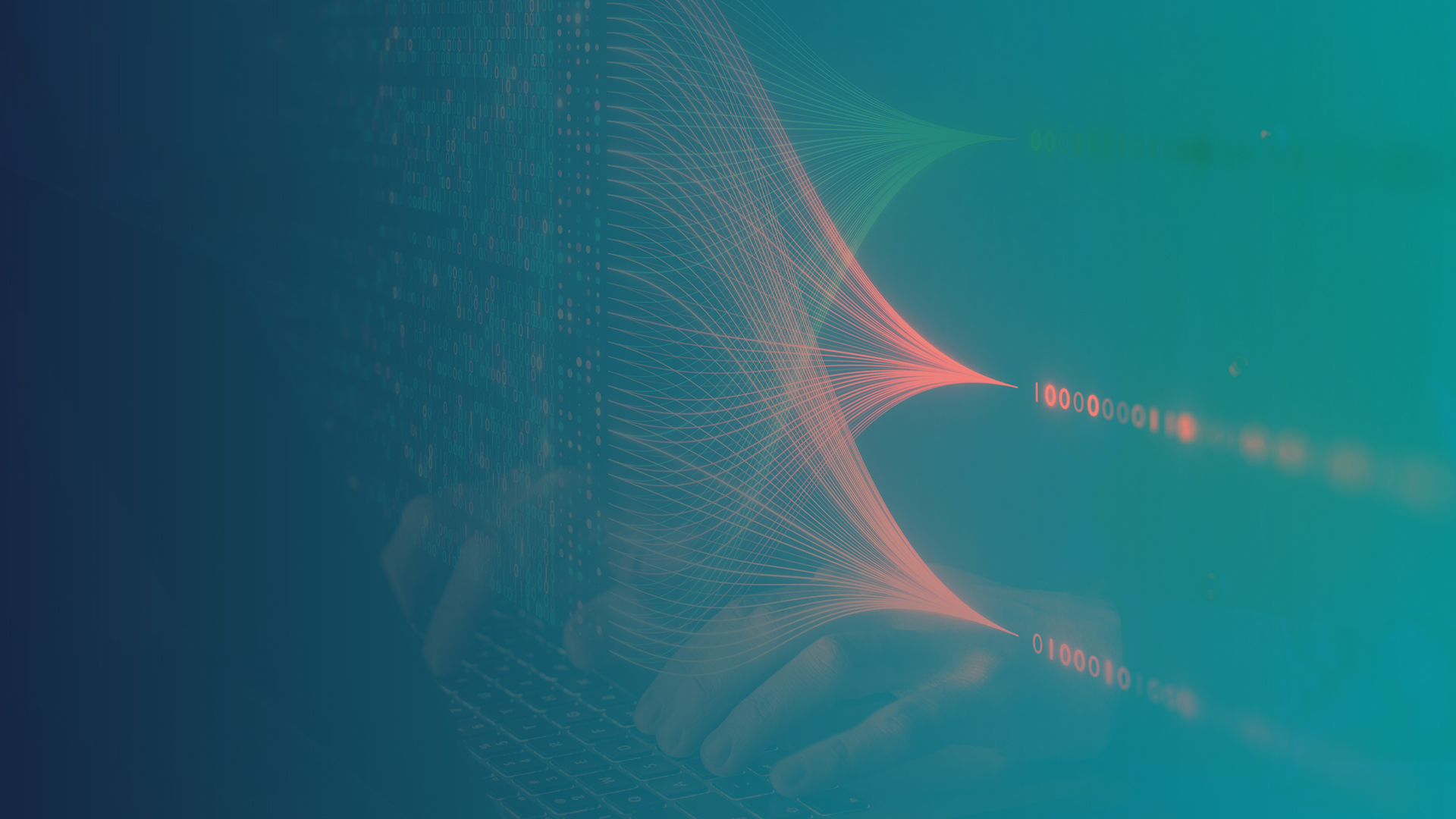
Data and analytics make the world go round
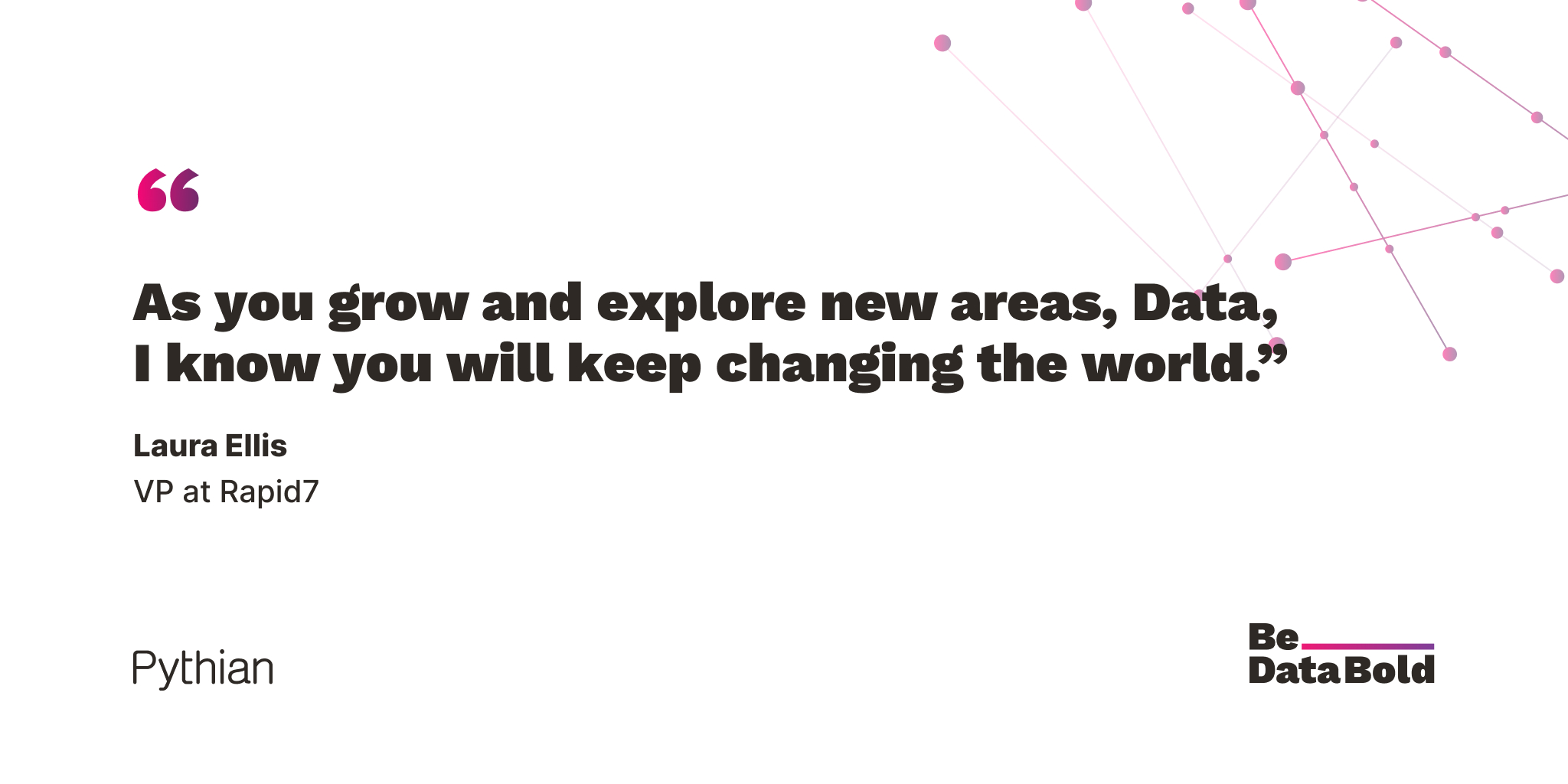
No Comments Yet
Let us know what you think